TensorFlow 入门之手写识别(MNIST) 数据处理 一
2017-10-19 10:13
375 查看
TensorFlow 入门之手写识别(MNIST) 数据处理 一
MNIST Fly softmax回归准备数据
解压 与 重构
手写识别入门MNIST手写数据集
图片以及标签的数据格式处理
准备数据
MNIST是在机器学习领域中的一个经典问题。该问题解决的是把28x28像素的灰度手写数字图片识别为相应的数字,其中数字的范围从0到9.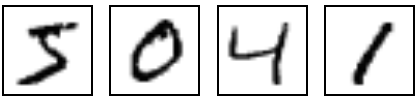
from IPython.display import Image
import base64
Image(data=base64.decodestring(url),embed=True)
同时我们可以通过TensorFlow提供的例子来下载有Yann LeCun提供的MNIST提供的如上的手写数据集。
文件 | 内容 |
---|---|
train-images-idx3-ubyte.gz | 训练集图片 - 55000 张 训练图片, 5000 张 验证图片 |
train-labels-idx1-ubyte.gz | 训练集图片对应的数字标签 |
t10k-images-idx3-ubyte.gz | 测试集图片 - 10000 张 图片 |
t10k-labels-idx1-ubyte.gz | 测试集图片对应的数字标签 |
import urllib
SOURCE_URL = 'http://yann.lecun.com/exdb/mnist/'
# WORK_DIRECTORY = "/tmp/mnist-data"
WORK_DIRECTORY = '/home/fly/TensorFlow/mnist'
def maybe_download(filename):
"""A helper to download the data files if not present."""
if not os.path.exists(WORK_DIRECTORY):
os.mkdir(WORK_DIRECTORY)
filepath = os.path.join(WORK_DIRECTORY, filename)
if not os.path.exists(filepath):
filepath, _ = urllib.urlretrieve(SOURCE_URL + filename, filepath)
statinfo = os.stat(filepath)
print 'Succesfully downloaded', filename, statinfo.st_size, 'bytes.'
else:
print 'Already downloaded', filename
return filepath
train_data_filename = maybe_download('train-images-idx3-ubyte.gz')
train_labels_filename = maybe_download('train-labels-idx1-ubyte.gz')
test_data_filename = maybe_download('t10k-images-idx3-ubyte.gz')
test_labels_filename = maybe_download('t10k-labels-idx1-ubyte.gz')
解压 与 重构
数据被解压成一个二维的Tensor:[image, index, pixel, index],pixel 列是像素的点。0表示的是背景色(白色),255表示的是前景色(黑色)。上面下载的数据是压缩的格式,我们需要解压它。而且每一幅图是值为[0...255],我们要将它们缩放到[-0.5, 0.5]之间。
我们可以来看一下图片解压后的文件格式:
[offset] [type] [value] [description] 0000 32 bit integer 0x00000803(2051) magic number 0004 32 bit integer 60000 number of images 0008 32 bit integer 28 number of rows 0012 32 bit integer 28 number of columns 0016 unsigned byte ?? pixel 0017 unsigned byte ?? pixel ........ xxxx unsigned byte ?? pixel
对应的代码是:
import gzip, binascii, struct, numpy
import matplotlibpyplot as plt
with gzip.open(test_data_filename) as f:
# 打印出解压后的图片格式的头格式
for field in ['magic number', 'image count', 'rows', 'columns']:
# struct.unpack reads the binary data provided by f.read.
# The format string '>i' decodes a big-endian integer, which
# is the encoding of the data.
print field, struct.unpack('>i', f.read(4))[0]
buf = f.read(28*28)
image = numpy.frombuffer(buf, dtype=numpy.uint8)
# 打印出前十个image的数据
print 'First 10 pixels: ', iamge[:10]
# ==>
# magic number 2051
# image count 10000
# rows 28
# columns 28
# First 10 pixels: [0 0 0 0 0 0 0 0 0 0]
当然我们也可以将解压后的图给绘制出来
# 我们将绘制图以及关于这个图的直方图
_, (ax1, ax2) = plt.subplots(1,2)
ax1.imshow(image.reshape(28,28), cmap=plt.cm.Greys)
ax2.hist(image, bins=20, range=[0,255])
我们也可以将reScale后的数据绘制出来看看
# Let's convert the uint8 image to 32 bit floats and rescale
# the values to be centered around 0, between [-0.5, 0.5].
#
# We again plot the image and histogram to check that we
# haven't mangled the data.
scaled = image.astype(numpy.float32)
scaled = (scaled - (255 / 2.0)) / 255
_, (ax1, ax2) = plt.subplots(1, 2)
ax1.imshow(scaled.reshape(28, 28), cmap=plt.cm.Greys);
ax2.hist(scaled, bins=20, range=[-0.5, 0.5]);
具体的数据使用可以看TensorFlow提供的测试代码[在IPython中是第三个实例3_mnist_from_scratch.ipynb]
手写识别入门
MNIST手写数据集
MNIST数据集的官网是Yann LeCun's website。 在这里,我们提供了一份python源代码用于自动下载和安装这个数据集。你可以下载这份代码,然后用下面的代码导入到你的项目里面,也可以直接复制粘贴到你的代码文件里面。(当然你也可以使用前面提到的代码来下载手写的数字数据)from tensorflow.examples.tutorials.mnist import input_data
# 通过指定下载地址就可以下载数据
mnist = input_data.read_data_sets("/home/fly/TensorFlow/mnist", one_hot=True)
图片以及标签的数据格式处理
下载解压后,得到的数据分为两部分,60000行的训练集(mnist.train)和10000行的测试数据集(mnist.test)。由前面的介绍可以知道,每个MNIST数据有两部分组成:一个手写数字的图片以及一个对应的标签。比如在训练集中数据图片为mnist.train.images以及标签mnist.train.labels.因为每一张图片是28 x 28的像素,所以我们可以使用一个数字数组来表示这张图:
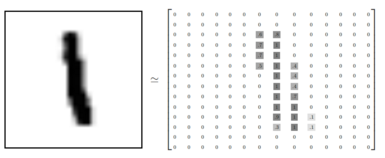
然后我们再把这个数组展开为长度为28 * 28 = 784 的 一维向量。因此,在MNIST训练数据集中,mnist.train.images 是一个形状为 [60000, 784] 的张量,第一个维度数字用来索引图片,第二个维度数字用来索引每张图片中的像素点。在此张量里的每一个元素,都表示某张图片里的某个像素的强度值,值介于0和1之间。
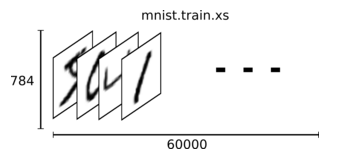
相对应的MNIST数据集的标签是介于0到9的数字,用来描述给定图片里表示的数字。比如,标签0将表示成([1,0,0,0,0,0,0,0,0,0,0]).因此, mnist.train.labels 是一个 [60000, 10] 的数字矩阵。

Fly
2016.6
相关文章推荐
- tensorflow的入门--手写数字识别mnist的傻瓜教程
- TensorFlow手写字识别mnist入门篇(代码及详解)
- TensorFlow 入门之手写识别(MNIST) softmax算法
- 基于tensorflow的MNIST手写数字识别--入门篇
- 30分钟手把手带你入门TensorFlow——Mnist手写数字识别实战教程
- [TensorFlow]入门学习笔记(2)-卷积神经网络mnist手写识别
- tensorflow入门实践例子—MNIST手写数字识别
- TensorFlow 入门之手写识别(MNIST) softmax算法 二
- tensorflow入门-mnist手写数字识别(一)
- tensorflow 入门小例子(mnist手写数字识别)
- 基于tensorflow的MNIST手写数字识别(二)--入门篇
- 基于tensorflow的MNIST手写数字识别(二)--入门篇
- 【Tensorflow入门】手写字体识别(MNIST)
- 用MXnet入门实战深度学习之一:安装GPU版mxnet并跑一个MNIST手写数字识别
- 46、tensorflow入门初步,手写识别0,1,2,3,4,5,6
- tensorflow构建RNN识别mnist手写数字
- 基于tensorflow的MNIST手写数字识别(三)--神经网络篇
- Tensorflow项目实战一:MNIST手写数字识别
- 用MXnet入门实战深度学习之一:安装GPU版mxnet并跑一个MNIST手写数字识别
- TensorFlow DNN 以库函数的方式实现MNIST手写识别