【机器学习实战二:朴素贝叶斯算法之过滤垃圾邮件】
2016-04-12 17:24
627 查看
http://blog.csdn.net/u013634684/article/details/49306563
标签: 机器学习python贝叶斯算法垃圾邮件过滤
2015-11-05 22:35 173人阅读 评论(0) 收藏 举报

分类:
机器学习(2)

版权声明:本文为博主原创文章,未经博主允许不得转载。
一、部分说明
----------------------------------------------------------------
1、本文代码是《机器学习实战》这本书的例程。点击下载《机器学习实战》及原书中的源代码;
2、运行环境为:window10+python3.2+各种python机器学习库。
安装可参考:点击打开链接
3、本文注重代码的实现过程,其基本知识请参考《机器学习实战》和一些知名博客。
4、本人属于初学者,有些注释部分可能不对,还请指正。
5、(1)、本代码从原来的python2.x改为python3.x;
(2)、加入详细的注释,方便理解;
(3)、还有加入自己想要实现的一些功能。
----------------------------------------------------------------
二、重点回顾
1、朴素贝叶斯算法原理:
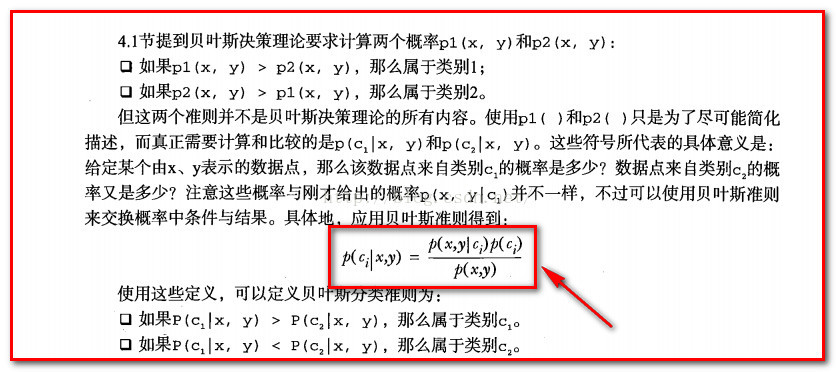
2、朴素贝叶斯算法原理用于垃圾邮件过滤:
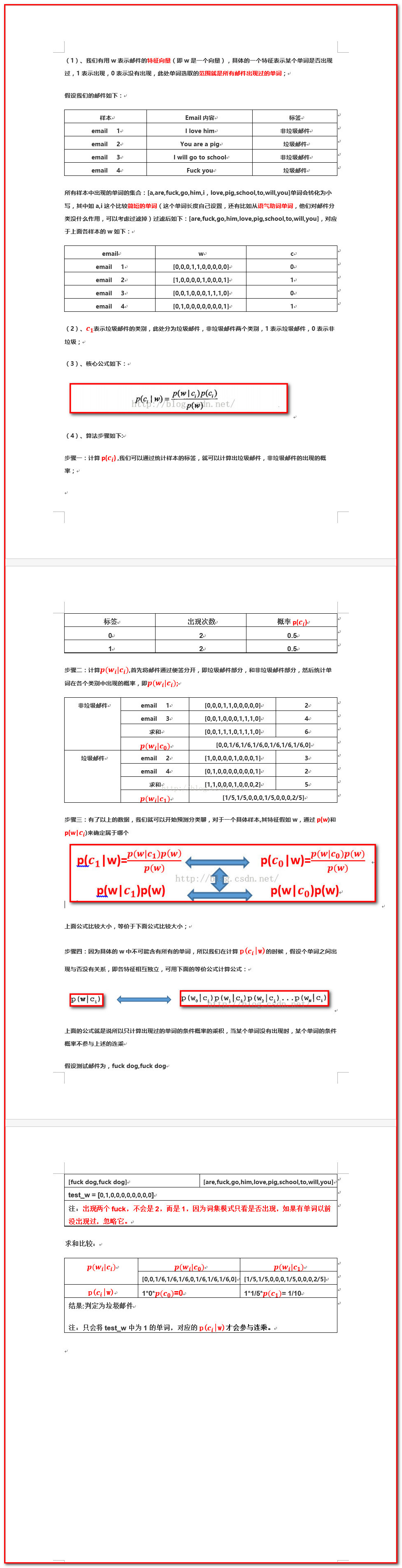
3、注意事项
(1)、为了防止步骤四的小数相乘造成精度损失(及比较小的数相乘最后会被当作0),故通过相乘比较大小转化为对数相加,避免此类问题的出现,影响实验结果;
(2)、分类时候还分为词集模式,知关心某个单词是否出现在邮件中;词袋模式,不仅关心单词是否出现还关心出现的次数;
(3)、其他一些具体的注意事项,会在代码中标注,可以查看,方便理解;
三、源代码
[python] view
plain copy
<strong>'''''***********************************************************
Copyright (C), 2015-2020, cyp Tech. Co., Ltd.
FileName: 朴素贝叶斯实例.cpp
Author: cyp
Version : v1.0
Date: 2015-10-21 上午10:38
Description: 利用朴素贝叶斯原理对邮件进行分类
Function List:
1. createVocalList:计算输入各集的并集,且每个元素是unique
2. setofWords2Vec: 运用词集方式将数据预处理
3. bagofWords2Vec: 运用词袋方式将数据预处理
4. trainNB0: 训练数据
5. classifyNB: 对email进行分类
6. test 相当于主函数,训练模型并测试
***********************************************************'''
'''''***********************************************************
需要导入的模块
***********************************************************'''
import random
import math
import numpy as np
import os
'''''***********************************************************
Function: createVocalList
Description: 计算输入各集的并集,且每个元素是unique
Calls:
Called By: test
Input: dataSet输入的数据集,及单词向量集
Return: 返回输入数据集的并集,返回数据的数据类型是list
表示出现过的单词
************************************************************'''
def createVocalList(dataSet):
vocalSet = set([]) #创建一个空集
for data in dataSet:
vocalSet = vocalSet | set(data) #利用set的特性求两个集合的并集
return list(vocalSet) #以list的方式返回结果
'''''***********************************************************
Function: setofWords2Vec
Description: 判断的向量的单词是否在词库中是否出现,如果出现
则标记为1,否则标记为0
Calls:
Called By: test
Input:
vocabList:
词库,曾经出现过的单词集合,即createVocalList
inputSet:
测试的单词向量
Return: returnVec是一个同vocalList同大小的数据,如果inputset单词
出现在vocalList则将returnVec对应的位置置1,否则为0
************************************************************'''
def setofWords2Vec(vocalList,inputset):
returnVec = [0]*len(vocalList)
#同vocalList同大小的list,开始假设每个单词都没出现,即全为0
for word in inputset:
if word in vocalList: #如果输入的单词出现在词库中,则置1
returnVec[vocalList.index(word)] = 1
else: #如果没出现在词库中,默认为0,并输出
print("the word:%s is not in my Vocabulary!"%word)
return returnVec #返回结果
'''''***********************************************************
Function: bagofWords2Vec
Description: 统计的单词是否在词库中出现频率
Calls:
Called By: test
Input:
vocabList:
词库,曾经出现过的单词集合,即createVocalList
inputSet:
测试的单词向量
Return: returnVec是一个同vocalList同大小的数据,对应位置
表示该单词出现的个数
************************************************************'''
def bagofWords2Vec(vocalList,inputset):
returnVec = [0]*len(vocalList)
#同vocalList同大小的list,开始假设每个单词都没出现,即全为0
for word in inputset:
if word in vocalList: #如果输入的单词出现在词库中,则加1
returnVec[vocalList.index(word)] += 1
return returnVec #返回结果
'''''***********************************************************
Function: trainNB0
Description: 统计侮辱性email出现概率,侮辱性邮件各单词出现的概率,非
侮辱性email各单词出现概率
Calls:
Called By: test
Input:
trainDataSet:参与训练的数据集,即setofWords2Vec返回数据的集合
trainLabels:训练数据的标签
Return:
pShame:侮辱性email
p0Vect:非侮辱性email中单词出现的概率
p1Vect:侮辱性email中单词出现的概率
************************************************************'''
def trainNB0(trainDataSet,trainLabels):
numTrains = len(trainDataSet) #训练数据的组数
numWords = len(trainDataSet[0]) #每组训练数据的大小
pShame = sum(trainLabels)/float(numTrains)
#标签中1表示侮辱,0表示非侮辱,故上述统计侮辱性email出现概率
#zeros(numWords)表示1*numWords数组,非numWords*numWords数组
#p0Num = np.zeros(numWords) #存储统计非侮辱性邮件各单词出现频率
#p1Num = np.zeros(numWords) #存储统计侮辱性邮件各单词出现频率
#p0SumWords = 0.0 #非侮辱性邮件中单词总数
#p1SumWords = 0.0 #侮辱性邮件中单词总数
#为了防止与0相乘,故初始化为1,因为两者的初始化一样,所以不影响结果
p0Num = np.ones(numWords) #存储统计非侮辱性邮件各单词出现频率
p1Num = np.ones(numWords) #存储统计侮辱性邮件各单词出现频率
p0SumWords = 2.0 #非侮辱性邮件中单词总数
p1SumWords = 2.0 #侮辱性邮件中单词总数
for i in range(numTrains):
if trainLabels[i]==1: #如果为侮辱性email
p1Num += trainDataSet[i] #统计非侮辱性邮件各单词
else:
p0Num += trainDataSet[i] #统计侮辱性邮件各单词
p0SumWords = sum(p0Num) #计算非侮辱性邮件中单词总数
p1SumWords = sum(p1Num) #计算侮辱性邮件中单词总数
p0Vect = p0Num/p0SumWords #非侮辱性邮件中单词出现的概率
p1Vect = p1Num/p1SumWords #侮辱性邮件中单词出现的概率
return pShame,p0Vect,p1Vect
'''''***********************************************************
Function: classifyNB
Description: 对email进行分类
Calls:
Called By: test
Input:
vec2Classify:要分类的数据
pShame:侮辱性email
p0Vect:非侮辱性email中单词出现的概率
p1Vect:侮辱性email中单词出现的概率
Return:
分类的结果,1表示侮辱性email,0表示非侮辱性email
************************************************************'''
def classifyNB(vec2Classify,p0Vect,p1Vect,pShame):
#由于小数相乘,会有很大误差,故先求对数再相乘
temp0 = vec2Classify*p0Vect
temp1 = vec2Classify*p1Vect
temp00 = []
temp11 = []
#分步求对数,因为log不能处理array,list
for x in temp0:
if x>0:
temp00.append(math.log(x))
else:
temp00.append(0)
for x in temp1:
if x>0:
temp11.append(math.log(x))
else:
temp11.append(0)
p0 = sum(temp00)+math.log(1-pShame) #属于非侮辱性email概率
p1 = sum(temp11)+math.log(pShame) #属于侮辱性email概率
if p1 > p0: #属于侮辱性email概率大于属于非侮辱性email概率
return 1
else:
return 0
'''''***********************************************************
Function: text2VecOfWords
Description: 从email的string中提取单词
Calls:
Called By: test
Input:
string: email字符串
Return:
单词向量
************************************************************'''
def text2VecOfWords(string):
import re #正则表达式工具
listOfWolds = re.split(r"\W*",string)
#分割数据,其分隔符是除单词,数字外任意的字符串
return [word.lower() for word in listOfWolds if len(word)>2]
#单词不区分大小写,及全部转换为(小写),滤除没用短字符串
'''''***********************************************************
Function: test
Description: 将数据部分用来训练模型,部分用来测试
Calls: text2VecOfWords
createVocalList
trainNB0
classifyNB
Called By: main
Input:
Return:
************************************************************'''
def test():
emailList = [] #存放每个邮件的单词向量
emailLabel = [] #存放邮件对应的标签
cwd = "C:\\Users\\cyp\\Documents\\sourcecode\\machinelearning\\my__code\\chapter4\\"
for i in range(1,26):
#读取非侮辱邮件,并生成单词向量
wordList = text2VecOfWords(open(cwd+r"email\ham\%d.txt"%i,encoding='Shift_JIS').read())
emailList.append(wordList)#将单词向量存放到emailList中
emailLabel.append(0) #存放对应的标签
wordList = text2VecOfWords(open(cwd+r"email\spam\%d.txt"%i,encoding='Shift_JIS').read())
#读取侮辱邮件,并生成单词向量
emailList.append(wordList)#将单词向量存放到emailList中
emailLabel.append(1) #存放对应的标签
vocabList = createVocalList(emailList)#由所有的单词向量生成词库
trainSet = [i for i in range(50)] #产生0-49的50个数字
testIndex = [] #存放测试数据的下标
for i in range(10): #从[0-49]中随机选取10个数
randIndex = int(random.uniform(0,len(trainSet)))
#随机生成一个trainSet的下标
testIndex.append(trainSet[randIndex])#提取对应的数据作为训练数据
del(trainSet[randIndex]) #删除trainSet对应的值,以免下次再选中
trainDataSet = [] #存放训练数据(用于词集方法)
trainLabels = [] #存放训练数据标签(用于词集方法)
trainDataSet1 = [] #存放训练数据(用于词袋方法)
trainLabels1 = [] #存放训练数据标签(用于词袋方法)
for index in trainSet: #trainSet剩余值为训练数据的下标
trainDataSet.append(setofWords2Vec(vocabList,emailList[index]))
#提取训练数据
trainLabels.append(emailLabel[index])
#提取训练数据标签
trainDataSet1.append(bagofWords2Vec(vocabList,emailList[index]))
#提取训练数据
trainLabels1.append(emailLabel[index])
#提取训练数据标签
pShame,p0Vect,p1Vect = trainNB0(trainDataSet,trainLabels)#开始训练
pShame1,p0Vect1,p1Vect1 = trainNB0(trainDataSet1,trainLabels1)#开始训练
errorCount = 0.0 #统计测试时分类错误的数据的个数
errorCount1= 0.0 #统计测试时分类错误的数据的个数
for index in testIndex:
worldVec = setofWords2Vec(vocabList,emailList[index])#数据预处理
#进行分类,如果分类不正确,错误个位数加1
if classifyNB(worldVec,p0Vect,p1Vect,pShame) != emailLabel[index]:
errorCount += 1
worldVec = bagofWords2Vec(vocabList,emailList[index])#数据预处理
#进行分类,如果分类不正确,错误个位数加1
if classifyNB(worldVec,p0Vect1,p1Vect1,pShame1) != emailLabel[index]:
errorCount1 += 1
#输出分类错误率
print("As to set,the error rate is :",float(errorCount)/len(testIndex))
print("AS to bag,the error rate is :",float(errorCount1)/len(testIndex))
'''''***********************************************************
Function: main
Description: 运行test函数
Calls: test
Called By:
Input:
Return:
************************************************************'''
if __name__=="__main__":
test()</strong>
[python] view
plain copy
<span style="font-family: Microsoft YaHei; font-size: 18px;"><strong>四、结果展示</strong></span>
标签: 机器学习python贝叶斯算法垃圾邮件过滤
2015-11-05 22:35 173人阅读 评论(0) 收藏 举报

分类:
机器学习(2)

版权声明:本文为博主原创文章,未经博主允许不得转载。
一、部分说明
----------------------------------------------------------------
1、本文代码是《机器学习实战》这本书的例程。点击下载《机器学习实战》及原书中的源代码;
2、运行环境为:window10+python3.2+各种python机器学习库。
安装可参考:点击打开链接
3、本文注重代码的实现过程,其基本知识请参考《机器学习实战》和一些知名博客。
4、本人属于初学者,有些注释部分可能不对,还请指正。
5、(1)、本代码从原来的python2.x改为python3.x;
(2)、加入详细的注释,方便理解;
(3)、还有加入自己想要实现的一些功能。
----------------------------------------------------------------
二、重点回顾
1、朴素贝叶斯算法原理:
2、朴素贝叶斯算法原理用于垃圾邮件过滤:
3、注意事项
(1)、为了防止步骤四的小数相乘造成精度损失(及比较小的数相乘最后会被当作0),故通过相乘比较大小转化为对数相加,避免此类问题的出现,影响实验结果;
(2)、分类时候还分为词集模式,知关心某个单词是否出现在邮件中;词袋模式,不仅关心单词是否出现还关心出现的次数;
(3)、其他一些具体的注意事项,会在代码中标注,可以查看,方便理解;
三、源代码
[python] view
plain copy
<strong>'''''***********************************************************
Copyright (C), 2015-2020, cyp Tech. Co., Ltd.
FileName: 朴素贝叶斯实例.cpp
Author: cyp
Version : v1.0
Date: 2015-10-21 上午10:38
Description: 利用朴素贝叶斯原理对邮件进行分类
Function List:
1. createVocalList:计算输入各集的并集,且每个元素是unique
2. setofWords2Vec: 运用词集方式将数据预处理
3. bagofWords2Vec: 运用词袋方式将数据预处理
4. trainNB0: 训练数据
5. classifyNB: 对email进行分类
6. test 相当于主函数,训练模型并测试
***********************************************************'''
'''''***********************************************************
需要导入的模块
***********************************************************'''
import random
import math
import numpy as np
import os
'''''***********************************************************
Function: createVocalList
Description: 计算输入各集的并集,且每个元素是unique
Calls:
Called By: test
Input: dataSet输入的数据集,及单词向量集
Return: 返回输入数据集的并集,返回数据的数据类型是list
表示出现过的单词
************************************************************'''
def createVocalList(dataSet):
vocalSet = set([]) #创建一个空集
for data in dataSet:
vocalSet = vocalSet | set(data) #利用set的特性求两个集合的并集
return list(vocalSet) #以list的方式返回结果
'''''***********************************************************
Function: setofWords2Vec
Description: 判断的向量的单词是否在词库中是否出现,如果出现
则标记为1,否则标记为0
Calls:
Called By: test
Input:
vocabList:
词库,曾经出现过的单词集合,即createVocalList
inputSet:
测试的单词向量
Return: returnVec是一个同vocalList同大小的数据,如果inputset单词
出现在vocalList则将returnVec对应的位置置1,否则为0
************************************************************'''
def setofWords2Vec(vocalList,inputset):
returnVec = [0]*len(vocalList)
#同vocalList同大小的list,开始假设每个单词都没出现,即全为0
for word in inputset:
if word in vocalList: #如果输入的单词出现在词库中,则置1
returnVec[vocalList.index(word)] = 1
else: #如果没出现在词库中,默认为0,并输出
print("the word:%s is not in my Vocabulary!"%word)
return returnVec #返回结果
'''''***********************************************************
Function: bagofWords2Vec
Description: 统计的单词是否在词库中出现频率
Calls:
Called By: test
Input:
vocabList:
词库,曾经出现过的单词集合,即createVocalList
inputSet:
测试的单词向量
Return: returnVec是一个同vocalList同大小的数据,对应位置
表示该单词出现的个数
************************************************************'''
def bagofWords2Vec(vocalList,inputset):
returnVec = [0]*len(vocalList)
#同vocalList同大小的list,开始假设每个单词都没出现,即全为0
for word in inputset:
if word in vocalList: #如果输入的单词出现在词库中,则加1
returnVec[vocalList.index(word)] += 1
return returnVec #返回结果
'''''***********************************************************
Function: trainNB0
Description: 统计侮辱性email出现概率,侮辱性邮件各单词出现的概率,非
侮辱性email各单词出现概率
Calls:
Called By: test
Input:
trainDataSet:参与训练的数据集,即setofWords2Vec返回数据的集合
trainLabels:训练数据的标签
Return:
pShame:侮辱性email
p0Vect:非侮辱性email中单词出现的概率
p1Vect:侮辱性email中单词出现的概率
************************************************************'''
def trainNB0(trainDataSet,trainLabels):
numTrains = len(trainDataSet) #训练数据的组数
numWords = len(trainDataSet[0]) #每组训练数据的大小
pShame = sum(trainLabels)/float(numTrains)
#标签中1表示侮辱,0表示非侮辱,故上述统计侮辱性email出现概率
#zeros(numWords)表示1*numWords数组,非numWords*numWords数组
#p0Num = np.zeros(numWords) #存储统计非侮辱性邮件各单词出现频率
#p1Num = np.zeros(numWords) #存储统计侮辱性邮件各单词出现频率
#p0SumWords = 0.0 #非侮辱性邮件中单词总数
#p1SumWords = 0.0 #侮辱性邮件中单词总数
#为了防止与0相乘,故初始化为1,因为两者的初始化一样,所以不影响结果
p0Num = np.ones(numWords) #存储统计非侮辱性邮件各单词出现频率
p1Num = np.ones(numWords) #存储统计侮辱性邮件各单词出现频率
p0SumWords = 2.0 #非侮辱性邮件中单词总数
p1SumWords = 2.0 #侮辱性邮件中单词总数
for i in range(numTrains):
if trainLabels[i]==1: #如果为侮辱性email
p1Num += trainDataSet[i] #统计非侮辱性邮件各单词
else:
p0Num += trainDataSet[i] #统计侮辱性邮件各单词
p0SumWords = sum(p0Num) #计算非侮辱性邮件中单词总数
p1SumWords = sum(p1Num) #计算侮辱性邮件中单词总数
p0Vect = p0Num/p0SumWords #非侮辱性邮件中单词出现的概率
p1Vect = p1Num/p1SumWords #侮辱性邮件中单词出现的概率
return pShame,p0Vect,p1Vect
'''''***********************************************************
Function: classifyNB
Description: 对email进行分类
Calls:
Called By: test
Input:
vec2Classify:要分类的数据
pShame:侮辱性email
p0Vect:非侮辱性email中单词出现的概率
p1Vect:侮辱性email中单词出现的概率
Return:
分类的结果,1表示侮辱性email,0表示非侮辱性email
************************************************************'''
def classifyNB(vec2Classify,p0Vect,p1Vect,pShame):
#由于小数相乘,会有很大误差,故先求对数再相乘
temp0 = vec2Classify*p0Vect
temp1 = vec2Classify*p1Vect
temp00 = []
temp11 = []
#分步求对数,因为log不能处理array,list
for x in temp0:
if x>0:
temp00.append(math.log(x))
else:
temp00.append(0)
for x in temp1:
if x>0:
temp11.append(math.log(x))
else:
temp11.append(0)
p0 = sum(temp00)+math.log(1-pShame) #属于非侮辱性email概率
p1 = sum(temp11)+math.log(pShame) #属于侮辱性email概率
if p1 > p0: #属于侮辱性email概率大于属于非侮辱性email概率
return 1
else:
return 0
'''''***********************************************************
Function: text2VecOfWords
Description: 从email的string中提取单词
Calls:
Called By: test
Input:
string: email字符串
Return:
单词向量
************************************************************'''
def text2VecOfWords(string):
import re #正则表达式工具
listOfWolds = re.split(r"\W*",string)
#分割数据,其分隔符是除单词,数字外任意的字符串
return [word.lower() for word in listOfWolds if len(word)>2]
#单词不区分大小写,及全部转换为(小写),滤除没用短字符串
'''''***********************************************************
Function: test
Description: 将数据部分用来训练模型,部分用来测试
Calls: text2VecOfWords
createVocalList
trainNB0
classifyNB
Called By: main
Input:
Return:
************************************************************'''
def test():
emailList = [] #存放每个邮件的单词向量
emailLabel = [] #存放邮件对应的标签
cwd = "C:\\Users\\cyp\\Documents\\sourcecode\\machinelearning\\my__code\\chapter4\\"
for i in range(1,26):
#读取非侮辱邮件,并生成单词向量
wordList = text2VecOfWords(open(cwd+r"email\ham\%d.txt"%i,encoding='Shift_JIS').read())
emailList.append(wordList)#将单词向量存放到emailList中
emailLabel.append(0) #存放对应的标签
wordList = text2VecOfWords(open(cwd+r"email\spam\%d.txt"%i,encoding='Shift_JIS').read())
#读取侮辱邮件,并生成单词向量
emailList.append(wordList)#将单词向量存放到emailList中
emailLabel.append(1) #存放对应的标签
vocabList = createVocalList(emailList)#由所有的单词向量生成词库
trainSet = [i for i in range(50)] #产生0-49的50个数字
testIndex = [] #存放测试数据的下标
for i in range(10): #从[0-49]中随机选取10个数
randIndex = int(random.uniform(0,len(trainSet)))
#随机生成一个trainSet的下标
testIndex.append(trainSet[randIndex])#提取对应的数据作为训练数据
del(trainSet[randIndex]) #删除trainSet对应的值,以免下次再选中
trainDataSet = [] #存放训练数据(用于词集方法)
trainLabels = [] #存放训练数据标签(用于词集方法)
trainDataSet1 = [] #存放训练数据(用于词袋方法)
trainLabels1 = [] #存放训练数据标签(用于词袋方法)
for index in trainSet: #trainSet剩余值为训练数据的下标
trainDataSet.append(setofWords2Vec(vocabList,emailList[index]))
#提取训练数据
trainLabels.append(emailLabel[index])
#提取训练数据标签
trainDataSet1.append(bagofWords2Vec(vocabList,emailList[index]))
#提取训练数据
trainLabels1.append(emailLabel[index])
#提取训练数据标签
pShame,p0Vect,p1Vect = trainNB0(trainDataSet,trainLabels)#开始训练
pShame1,p0Vect1,p1Vect1 = trainNB0(trainDataSet1,trainLabels1)#开始训练
errorCount = 0.0 #统计测试时分类错误的数据的个数
errorCount1= 0.0 #统计测试时分类错误的数据的个数
for index in testIndex:
worldVec = setofWords2Vec(vocabList,emailList[index])#数据预处理
#进行分类,如果分类不正确,错误个位数加1
if classifyNB(worldVec,p0Vect,p1Vect,pShame) != emailLabel[index]:
errorCount += 1
worldVec = bagofWords2Vec(vocabList,emailList[index])#数据预处理
#进行分类,如果分类不正确,错误个位数加1
if classifyNB(worldVec,p0Vect1,p1Vect1,pShame1) != emailLabel[index]:
errorCount1 += 1
#输出分类错误率
print("As to set,the error rate is :",float(errorCount)/len(testIndex))
print("AS to bag,the error rate is :",float(errorCount1)/len(testIndex))
'''''***********************************************************
Function: main
Description: 运行test函数
Calls: test
Called By:
Input:
Return:
************************************************************'''
if __name__=="__main__":
test()</strong>
[python] view
plain copy
<span style="font-family: Microsoft YaHei; font-size: 18px;"><strong>四、结果展示</strong></span>
相关文章推荐
- View的事件体系——View的位置参数
- tomcat7用jndi配置数据源出现java.lang.NoSuchMethodException: org.apache.catalina.deploy.WebXml addResourceRef
- Tyvj_P1004
- 【原创】k8s源码分析-----kubelet(6)statusManager
- SQL多表连接查询(详细实例)
- 正则表达式验证生日手机号信息
- C语言中的位操作
- I/O重定向:改变了数据的输出或输入来源
- 第七周上机实践项目2:友元类
- Java集合之ArrayList和LinkedList的实现原理以及Iterator详解
- Frozen your python script with PyInstaller - Linux Instance
- CRT session密码反解
- Tricks(三十三)—— 自定义 zipWithIndex
- Uploadify传参方式
- 关于Android,我的面试总结
- android AsyncQueryHandler的分析
- 高斯处理图像背景模糊
- 彭鑫博士:测测你阳气足不足
- IOS基础:深入理解Objective-c中@class的含义
- StarUML中时序图添加小人