数据挖掘笔记-情感倾向点互信息算法
2014-10-04 12:28
441 查看
点间互信息(PMI)主要用于计算词语间的语义相似度,基本思想是统计两个词语在文本中同时出现的概率,如果概率越大,其相关性就越紧密,关联度越高。两个词语word1与word2的PMI值计算公式如下式所示为:

P(word1&word2)表示两个词语word1与word2共同出现的概率,即word1与word2共同出现的文档数, P(word1)与P(word2)分别表示两个词语单独出现的概率,即word出现的文档数。若两个词语在数据集的某个小范围内共现概率越大,表明其关联度越大;反之,关联度越小。P(word1&word2)与P(word1)P(word2)的比值是word1与word2两个词语的统计独立性度量。其值可以转化为3
种状态:
P(word1&word2) > 0;两个词语是相关的;值越大,相关性越强。
P(word1&word2) = 0;两个词语是统计独立的,不相关也不互斥。
P(word1&word2) < 0;两个词语是不相关的,互斥的。
情感倾向点互信息算法(Semantic Orientation Pointwise Mutual Information, SO-PMI)是将PMI方法引入计算词语的情感倾向(Semantic
Orientation,简称SO)中,从而达到捕获情感词的目地。基于点间互信息SO-PMI 算法的基本思想是:首先分别选用一组褒义词跟一组贬义词作为基准词,假设分别用Pwords与Nwords来表示这两组词语。这些情感词必须是倾向性非常明显,而且极具领域代表性的词语。若把一个词语word1跟Pwords的点间互信息减去word1跟Nwords的点间互信息会得到一个差值,就可以根据该差
值判断词语word1的情感倾向。其计算公式如下式所示:
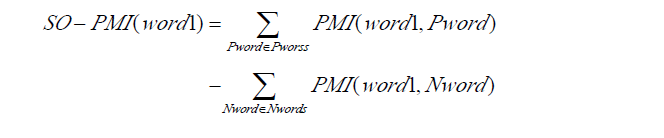
通常情况下,将0作为SO-PMI 算法的阀值。由此可以将得到三种状态:
SO-PMI(word1) > 0;为正面倾向,即褒义词
SO-PMI(word1) = 0;为中性倾向,即中性词
SO-PMI(word1) < 0;为负面倾向,即贬义词
下面是用Java简单实现情感倾向点互信息算法的一个例子:
代码托管:https://github.com/fighting-one-piece/repository-datamining.git
P(word1&word2)表示两个词语word1与word2共同出现的概率,即word1与word2共同出现的文档数, P(word1)与P(word2)分别表示两个词语单独出现的概率,即word出现的文档数。若两个词语在数据集的某个小范围内共现概率越大,表明其关联度越大;反之,关联度越小。P(word1&word2)与P(word1)P(word2)的比值是word1与word2两个词语的统计独立性度量。其值可以转化为3
种状态:
P(word1&word2) > 0;两个词语是相关的;值越大,相关性越强。
P(word1&word2) = 0;两个词语是统计独立的,不相关也不互斥。
P(word1&word2) < 0;两个词语是不相关的,互斥的。
情感倾向点互信息算法(Semantic Orientation Pointwise Mutual Information, SO-PMI)是将PMI方法引入计算词语的情感倾向(Semantic
Orientation,简称SO)中,从而达到捕获情感词的目地。基于点间互信息SO-PMI 算法的基本思想是:首先分别选用一组褒义词跟一组贬义词作为基准词,假设分别用Pwords与Nwords来表示这两组词语。这些情感词必须是倾向性非常明显,而且极具领域代表性的词语。若把一个词语word1跟Pwords的点间互信息减去word1跟Nwords的点间互信息会得到一个差值,就可以根据该差
值判断词语word1的情感倾向。其计算公式如下式所示:
通常情况下,将0作为SO-PMI 算法的阀值。由此可以将得到三种状态:
SO-PMI(word1) > 0;为正面倾向,即褒义词
SO-PMI(word1) = 0;为中性倾向,即中性词
SO-PMI(word1) < 0;为负面倾向,即贬义词
下面是用Java简单实现情感倾向点互信息算法的一个例子:
public class SemanticOrientationPointMutualInformation { //褒义词 private static Set<String> commendatories = null; //贬义词 private static Set<String> derogratories = null; static { commendatories = new HashSet<String>(); String commendatoryStr = "英俊 美丽 优雅 活泼 时尚 聪明 能干 健康 勤劳 阳光 好学 俏丽 忠心 善良 坚强 独立 团结 优美 义气 智慧 大度 豁达 开朗 富有 专心 勤劳 乐观 可爱 热心 孝顺 妩媚 丽人 矜持 佳丽 柔美 婉丽 娉婷 婉顺 娇柔 可爱 温柔 体贴 贤惠 贤慧 才干 人才 袅娜 赞美 赞赏"; StringTokenizer tokenizer = new StringTokenizer(commendatoryStr); while (tokenizer.hasMoreTokens()) { commendatories.add(tokenizer.nextToken()); } derogratories = new HashSet<String>(); String derogratoryStr = "矮小 猥琐 萎靡 奸诈 歹毒 毒辣 丑陋 弱智 愚笨 愚蠢 阴暗 贬斥 否定 憎恨 轻蔑 责骂 叛逆 汉奸 低能 恶心 阴险 白痴 变态 三八 腐败 呆板 呆滞 土气 无能 懒惰 慵懒 庸才 废物 风骚 下贱 病夫 脆弱 俗气 小气 贫穷 贫贱 花心 悲观 市井 小人 幼稚"; tokenizer = new StringTokenizer(derogratoryStr); while (tokenizer.hasMoreTokens()) { derogratories.add(tokenizer.nextToken()); } } //初始化文本 private DocumentSet initData() { DocumentSet documentSet = null; try { String path = SemanticOrientationPointMutualInformation.class.getClassLoader().getResource("微测").toURI().getPath(); documentSet = DocumentLoader.loadDocumentSetByThread(path); reduceDimensionsByCHI(documentSet); } catch (URISyntaxException e) { e.printStackTrace(); } return documentSet; } //开方检验特征选择降维 public void reduceDimensionsByCHI(DocumentSet documentSet) { IFeatureSelect featureSelect = new FSChiSquare(); featureSelect.handle(documentSet); List<Document> documents = documentSet.getDocuments(); for (Document document : documents) { Map<String, Double> chiWords = document.getChiWords(); List<Map.Entry<String, Double>> list = sortMap(chiWords); int len = list.size() < 100 ? list.size() : 100; String[] words = new String[len]; for (int i = 0; i < len; i++) { words[i] = list.get(i).getKey(); } document.setWords(words); } } public List<Map.Entry<String, Double>> sortMap(Map<String, Double> map) { List<Map.Entry<String, Double>> list = new ArrayList<Map.Entry<String, Double>>(map.entrySet()); Collections.sort(list, new Comparator<Map.Entry<String, Double>>() { @Override public int compare(Entry<String, Double> o1, Entry<String, Double> o2) { if (o1.getValue().isNaN()) { o1.setValue(0.0); } if (o2.getValue().isNaN()) { o2.setValue(0.0); } return -o1.getValue().compareTo(o2.getValue()); } }); return list; } /** * 计算点间互信息 * @param word1 * @param word2 * @param documents * @return */ private double calculatePMI(String word1, String word2, List<Document> documents) { double w1w2Num = statisticsWordsInDocs(documents, word1, word2); if (w1w2Num == 0) return 0; double w1Num = statisticsWordsInDocs(documents, word1); double w2Num = statisticsWordsInDocs(documents, word2); return Math.log(documents.size() * w1w2Num / (w1Num * w2Num)) / Math.log(2); } /** * 统计词出现文档数 * @param documents * @param words * @return */ private double statisticsWordsInDocs(List<Document> documents, String... words) { double sum = 0; for (Document document : documents) { Set<String> wordSet = document.getWordSet(); int num = 0; for (String word : words) { if (wordSet.contains(word)) num++; } if (num == words.length) sum++; } return sum; } public int judgeEmotion(double value) { return value == 0 ? Emotion.NEUTRAL : (value > 0 ? Emotion.COMMENDATORY : Emotion.DEROGRATORY); } public void build() { DocumentSet documentSet = initData(); Set<String> cs = new HashSet<String>(); Set<String> ds = new HashSet<String>(); List<Document> documents = documentSet.getDocuments(); for (Document document : documents) { String[] words = document.getWords(); for (String word : words) { double c = 0.0; for (String commendatory : commendatories) { c += calculatePMI(word, commendatory, documents); } if (c != 0) System.out.println("c: " + c); double d = 0.0; for (String derogratory : derogratories) { d += calculatePMI(word, derogratory, documents); } double value = c - d; if (value != 0) System.out.println("value: " + value); if (value > 0) { cs.add(word); } else if (value < 0) { ds.add(word); } } } System.out.println(cs); System.out.println(ds); } public static void main(String[] args) { new SemanticOrientationPointMutualInformation().build(); System.exit(0); } }
代码托管:https://github.com/fighting-one-piece/repository-datamining.git
相关文章推荐
- 数据挖掘笔记-情感倾向点互信息算法
- 数据挖掘笔记-分类-回归算法-最小二乘法
- 数据挖掘学习笔记——十大算法之决策树算法、逻辑回归概述
- 大数据学习笔记之四十一 数据挖掘算法之预测建模的回归模型
- 数据挖掘笔记-分类-回归算法-梯度上升
- 【数据挖掘】FPgrowth算法笔记
- 大数据学习笔记之三十八 数据挖掘算法之聚类分析
- 数据挖掘任务常用算法笔记
- 挖掘DBLP作者合作关系,FP-Growth算法实践(1):从DBLP数据集中提取目标信息(会议、作者等)
- 数据挖掘-关联规则挖掘理论和算法学习笔记
- 数据挖掘算法--分类与预测笔记
- 数据挖掘笔记-文本情感简单判断
- 数据挖掘笔记-特征选择-算法实现-1
- 【数据挖掘】算法学习笔记
- 大数据学习笔记之四十 数据挖掘算法之预测建模关于决策树模型的介绍
- 大数据学习笔记之三十七 数据挖掘算法之关联分析
- 大数据学习笔记之三十九 数据挖掘算法之预测建模
- 【数据挖掘】关联挖掘算法+信息增益等相关概念
- [数据挖掘课程笔记]基于规则的分类-顺序覆盖算法(sequential covering algorithm)
- 数据挖掘笔记-特征选择-算法实现-1