Non-local Sparse Models for Image Restoration
2013-08-09 16:28
543 查看
Non-local Sparse Models for Image Restoration
To_捭阖_youth
本文中作者提出了一种图像恢复算法,该算法的主要思想是确保图像的相似的图像块在同一个字典下的稀疏表示系数也是相似的,这样可以有效地恢复图像的结构信息,将 the non-local means 和 sparse coding 结合组成一个新的联合框架,相似块可以用similar sparse patterns 来分解,实验结果也进一步证明该算法在图像去燥和图像去马赛克方面取得比现有方法更好的结果。
Abstract
本文中提出两种图像恢复的方法:
(1)learning a basis set adapted to sparse signal descriptions
(2)exploiting the self-similarities of natural images and non-local means approach
上述通过jointly decomposing groups of similar signals on subsets of the learned dictionary可以得到。
Introduction
Early work:
various smoothness assumptions -----nisotropic filtering(各项异性滤波);total variation;image decompositions on fixed bases such as wavelets
non-local means filtering;image self-similarities;learned sparse models;Gaussian scale mixtures;fields of experts;block matching with 3D filtering(BM3D)
In this paper:
①explicitly exploits self-similarities in natural images to average out the noise among similar patches.
②sparse coding encodes natural image statistics by decomposing each image patch into a linear combination of a few elements from a basis set called a dictionary.
using simultaneous sparse coding to impose that similar patches share the same dictionary elements in their sparse decomposition.
本文与BM3D不一样的是BM3D基于典型固定正交字典。
Related Work
1、Non-Local Means Filtering
Reference:《a non-local algorithm for image denoising》
已知一个离散含噪图像v
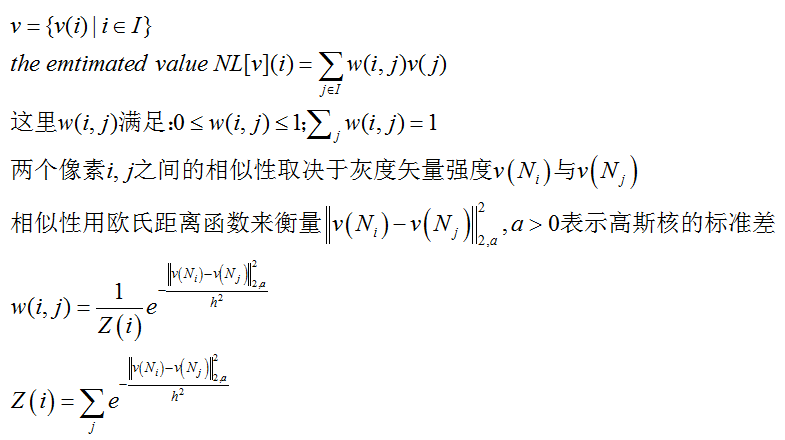
2、Learned Sparse Coding
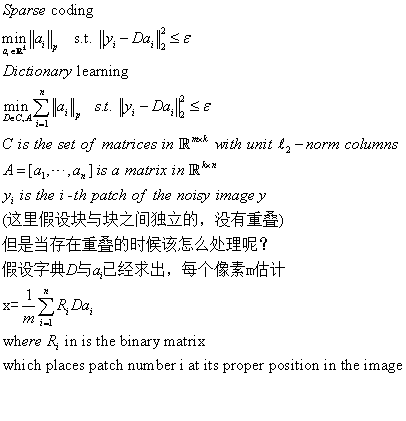
3、Block Matching 3D (BM3D)
Reference:《Image Denoising by Sparse 3-D Transform-Domain Collaborative Filtering》
简单来说类似于non-local means filtering,通过找到相似块来重构块,具体操作是把块堆积成3D的信号块再利用3D正交字典来hard or soft thresholding从而对块去噪。
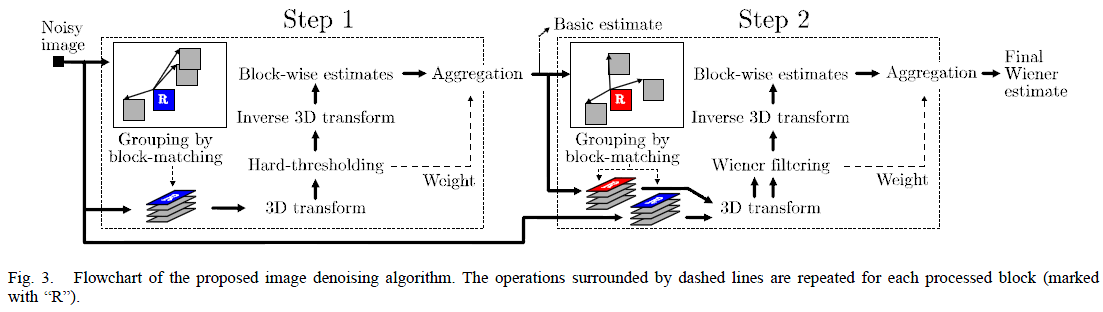
Proposed Formulation
该部分主要讲图像自相似性怎么融合到稀疏编码中???
1、Simultaneous Sparse Coding
由于稀疏分解潜在的不稳定性(0范数实际上是分段常数,1范数实际上是分段可微)稀疏编码太自由,相似块有时可能有不同的估计;故我们迫使相似块有相似分解。
联合稀疏表示模式指的就是一群非零的系数通过对矩阵(grouped-sparsity regularizer)分组稀疏操作强加到一组向量中;目的就是限制A非零行的数目。
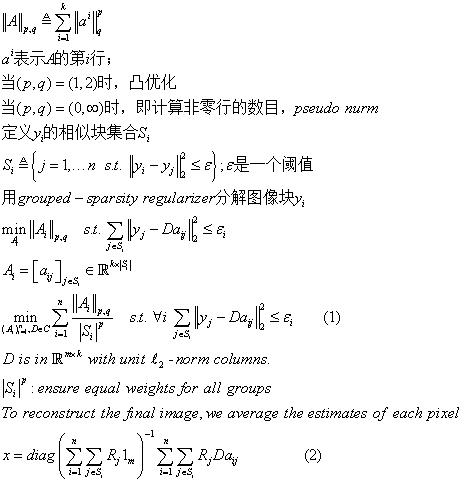
注意这里有一个问题:怎么确定(p,q)的值?
Reference:《Image denoising via sparse and redundant representations over learned dictionaries》
上文中讲到典型的字典学习中,我们常常采用1范数学习字典,采用0范数来进行图像最后的重构。
所以,这里我们采用(p,q)=(1,2)范数学习字典,(p,q)=(0,∞)范数来进行最后的重构。
2、Practical Formulation and Implementation
我们知道上述优化问题的计算量主要在于向量a的n*n的计算量,下面我们一下方法就是为了使这计算量对于n线性化并且最优化。
2.1、Semi-local grouping
Reference:《Image Denoising by Sparse 3-D Transform-Domain Collaborative Filtering》
建立w*w的搜索窗口来寻找y的相似块,这样可以将计算量减少为nw*w。
2.2、Clustering
首先把所有像素聚类到不相交的Ck,使Ck中所有的像素点i都有相同的Si集合。
在同一类所有像素点的优化问题是一致的,从而降低了整个计算量。实际上,因为每个像素点都有一个类,故只有n vertors a 被计算。
2.3、Initialization of D
Reference:《Online dictionary learning for sparse coding》
从PASCAL VOC‘07 数据库中随机选出10000副图像,从中取2*10^7图像块来学习字典。
2.4、Improved matching
我们发现在分组之前对图像简单的稀疏编码去噪对于相似块的分组很有帮助。
2.5、Patch normalization
为了提高稀疏编码的稳定性,我们一般在对图像块操作之前,图像块所有像素值都减去该块的平均强度(RGB 颜色),之后在操作结束后在估计值上加上这个平均强度。
其实这种方法应用真的很常见,一般去噪和超分辨(杨建超也就这样做的),这样可以提高结果的视觉效果。
3、Real Image and Demosaiking
本文去马赛克分为四步:
(1)Cluster similar patches on the mosaicked image y
(2)用D0重构每一块图像

(3)学习一个字典D1,对式子(1)最优化即可求解
(4)用D2=[D0 D1]代替式子(3)中D0,来重构每一块图像,之后用(2)式平均估计值最终得到整个去马赛克的图像。
Experimental Validation
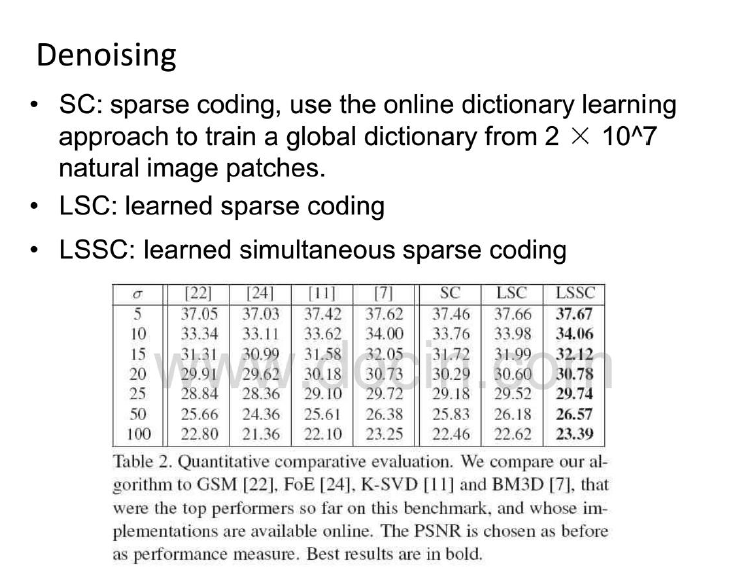
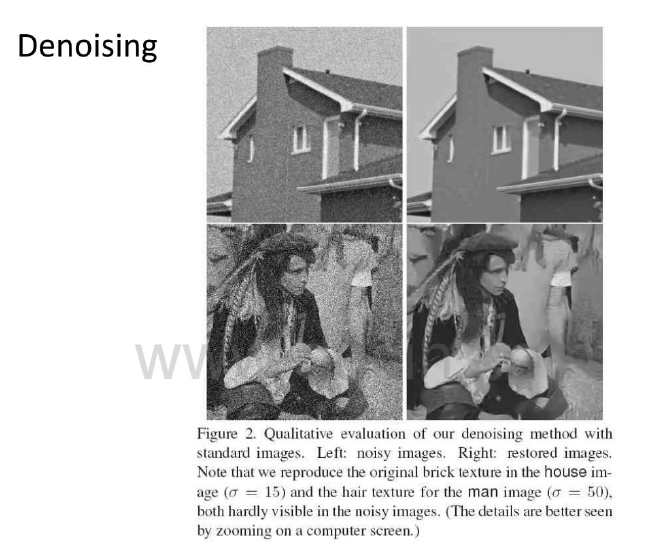
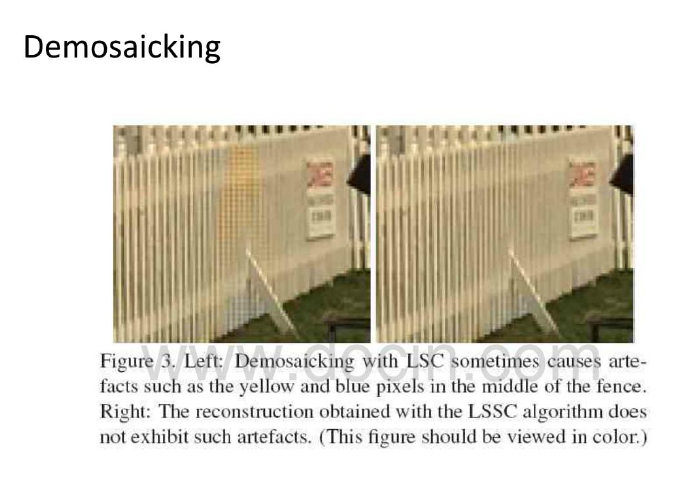
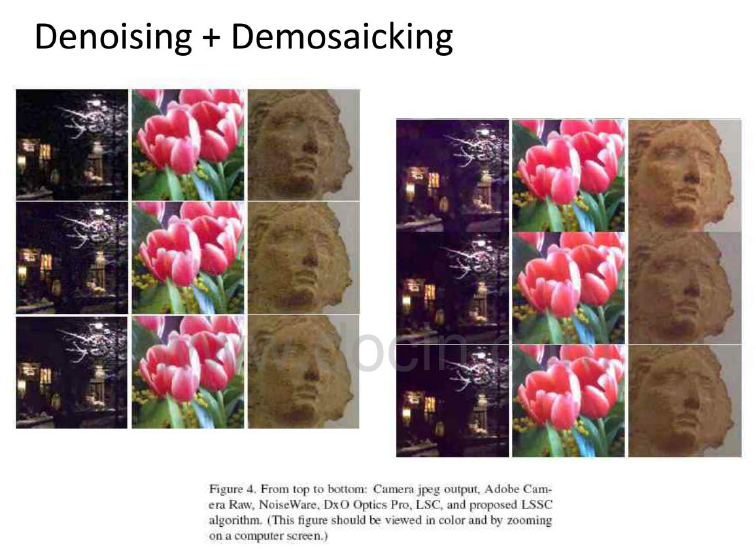
d
Download: Reference
本文尚不成熟,希望大家提出宝贵意见。
敬请关注本博客和新浪微博To_捭阖_youth.
To_捭阖_youth
本文中作者提出了一种图像恢复算法,该算法的主要思想是确保图像的相似的图像块在同一个字典下的稀疏表示系数也是相似的,这样可以有效地恢复图像的结构信息,将 the non-local means 和 sparse coding 结合组成一个新的联合框架,相似块可以用similar sparse patterns 来分解,实验结果也进一步证明该算法在图像去燥和图像去马赛克方面取得比现有方法更好的结果。
Abstract
本文中提出两种图像恢复的方法:
(1)learning a basis set adapted to sparse signal descriptions
(2)exploiting the self-similarities of natural images and non-local means approach
上述通过jointly decomposing groups of similar signals on subsets of the learned dictionary可以得到。
Introduction
Early work:
various smoothness assumptions -----nisotropic filtering(各项异性滤波);total variation;image decompositions on fixed bases such as wavelets
non-local means filtering;image self-similarities;learned sparse models;Gaussian scale mixtures;fields of experts;block matching with 3D filtering(BM3D)
In this paper:
①explicitly exploits self-similarities in natural images to average out the noise among similar patches.
②sparse coding encodes natural image statistics by decomposing each image patch into a linear combination of a few elements from a basis set called a dictionary.
using simultaneous sparse coding to impose that similar patches share the same dictionary elements in their sparse decomposition.
本文与BM3D不一样的是BM3D基于典型固定正交字典。
Related Work
1、Non-Local Means Filtering
Reference:《a non-local algorithm for image denoising》
已知一个离散含噪图像v
2、Learned Sparse Coding
3、Block Matching 3D (BM3D)
Reference:《Image Denoising by Sparse 3-D Transform-Domain Collaborative Filtering》
简单来说类似于non-local means filtering,通过找到相似块来重构块,具体操作是把块堆积成3D的信号块再利用3D正交字典来hard or soft thresholding从而对块去噪。
Proposed Formulation
该部分主要讲图像自相似性怎么融合到稀疏编码中???
1、Simultaneous Sparse Coding
由于稀疏分解潜在的不稳定性(0范数实际上是分段常数,1范数实际上是分段可微)稀疏编码太自由,相似块有时可能有不同的估计;故我们迫使相似块有相似分解。
联合稀疏表示模式指的就是一群非零的系数通过对矩阵(grouped-sparsity regularizer)分组稀疏操作强加到一组向量中;目的就是限制A非零行的数目。
注意这里有一个问题:怎么确定(p,q)的值?
Reference:《Image denoising via sparse and redundant representations over learned dictionaries》
上文中讲到典型的字典学习中,我们常常采用1范数学习字典,采用0范数来进行图像最后的重构。
所以,这里我们采用(p,q)=(1,2)范数学习字典,(p,q)=(0,∞)范数来进行最后的重构。
2、Practical Formulation and Implementation
我们知道上述优化问题的计算量主要在于向量a的n*n的计算量,下面我们一下方法就是为了使这计算量对于n线性化并且最优化。
2.1、Semi-local grouping
Reference:《Image Denoising by Sparse 3-D Transform-Domain Collaborative Filtering》
建立w*w的搜索窗口来寻找y的相似块,这样可以将计算量减少为nw*w。
2.2、Clustering
首先把所有像素聚类到不相交的Ck,使Ck中所有的像素点i都有相同的Si集合。
在同一类所有像素点的优化问题是一致的,从而降低了整个计算量。实际上,因为每个像素点都有一个类,故只有n vertors a 被计算。
2.3、Initialization of D
Reference:《Online dictionary learning for sparse coding》
从PASCAL VOC‘07 数据库中随机选出10000副图像,从中取2*10^7图像块来学习字典。
2.4、Improved matching
我们发现在分组之前对图像简单的稀疏编码去噪对于相似块的分组很有帮助。
2.5、Patch normalization
为了提高稀疏编码的稳定性,我们一般在对图像块操作之前,图像块所有像素值都减去该块的平均强度(RGB 颜色),之后在操作结束后在估计值上加上这个平均强度。
其实这种方法应用真的很常见,一般去噪和超分辨(杨建超也就这样做的),这样可以提高结果的视觉效果。
3、Real Image and Demosaiking
本文去马赛克分为四步:
(1)Cluster similar patches on the mosaicked image y
(2)用D0重构每一块图像
(3)学习一个字典D1,对式子(1)最优化即可求解
(4)用D2=[D0 D1]代替式子(3)中D0,来重构每一块图像,之后用(2)式平均估计值最终得到整个去马赛克的图像。
Experimental Validation
d
Download: Reference
本文尚不成熟,希望大家提出宝贵意见。
敬请关注本博客和新浪微博To_捭阖_youth.
相关文章推荐
- A non local algorithm for image denoising
- Hyperspectral image super-resolution via non-local sparse tensor factorization
- A non-local algorithm for image denoising
- Incoherent dictionary learning for sparse representation based image denoising(一)
- Why L1 norm for sparse models?
- [导入]generic file and image models for uploaded files
- 论文笔记之:Let there be Color!: Joint End-to-end Learning of Global and Local Image Priors for Automatic
- 关于2009_CVPR_Linear spatial pyramid matching using sparse coding for image classification
- (转) RNN models for image generation
- 论文阅读-Learning Deep CNN Denoiser Prior for Image Restoration
- Discriminative Learned Dictionaries for Local Image Analysis学习笔记
- 《Close the Loop: Joint Blind Image Restoration and Recognition with Sparse Representation Prior》阅读笔记
- Are sparse representations really relevant for image classification?
- Let there be Color!: Joint End-to-end Learning of Global and Local Image Priors for Automatic Image
- Non-Photorealistic Rendering (Domain transform for edge-aware image and video processing)
- Aggregating local features for Image Retrieval
- Passing address of non-local object to __autoreleasing parameter for write-back
- Exploring Models and Data for Image Question Answering
- 论文笔记之:Let there be Color!: Joint End-to-end Learning of Global and Local Image Priors for Automatic
- Linear Spatial Pyramid Matching Using Sparse Coding for Image Classification代码解